Application Architecture
Modern application architecture can be thought of as a secure data distribution problem, not just to and from the edge but extending all the way to real people and connected devices. Applications serving smaller user populations or with less data in motion can more easily meet their requirements, but as they grow, they put stress on different parts of the application architecture that need to scale effectively and efficiently.
Apache Kafka has emerged as a popular way to represent data as named streams of messages, called topics, with consumers that can track their progress and be resilient to failure, transparently recovering from where they left off. This has unlocked a different breed of time-decoupled and event-driven microservices.
Apache Kafka is designed to handle "dumb pipes" of messages really well, placing responsibility for the intelligence at the Kafka clients, but many clients are not directly compatible with the Kafka protocol. For example, HTML5 web clients understand HTTP, Server-Sent Events and WebSockets, while IoT clients often understand MQTT v3 and MQTT v5.
This raises the question of how web, mobile, and IoT clients should participate in modern application architectures centered on Apache Kafka.
Zilla sets out to make Apache Kafka feel natural to clients, using their own native protocols and SDKs while placing the power in the hands of DevOps to define their application-specific APIs to Apache Kafka.
The Zilla Proxy
Zilla strives to overcome many of the challenges of building a secure, high performance gateway. Low level optimizations can often force breaking an abstraction, whereas the modularity required by extensibility depends on keeping specific abstractions in place. Zilla strikes a balance between these opposing design constraints. For example, Zilla aligns with modern massively multi-core CPU architectures, while still retaining extensibility to support a diverse set of client-native protocols.
One common approach used by several Java based gateway architectures is to build on top of a protocol pipeline codec framework such as Apache MINA or the Netty project. However, these frameworks can encourage a significant amount of object allocation on the pipeline during protocol encoding from objects to bytes or decoding from bytes to objects.
We learned this based on first hand experience from implementing protocol servers in the past where garbage collection pauses could introduce unpredictable latency, and deep method call stacks could prevent the JavaVM from making JIT compiler optimizations such as method inlining or object escape analysis.
In contrast, Zilla uses code generated flyweight objects to overlay strongly typed APIs over raw binary data, without incurring any object allocation overhead, while isolating code into Java modules and keeping method call stacks short to help unlock JIT optimizations.
The Zilla engine is modular, scalable, and observable by design.
Modularity
The modules within Zilla cover each extensible aspect of the engine.
- bindings - represent each different stage in the protocol pipeline, including protocol servers,protocol clients, protocol mappings, and protocol-specific caches
- catalogs - retrieve API specifications to retrieve message type definitions
- vaults - securely store private keys and public certificates
- guards - verify trusted identity and enforce fine-grained access control
- models - enforce message validation or support message format conversion
- metrics - track generic or protocol-specific telemetry measurements
- exporters - integrate telemetry logs and metrics with external systems

Zilla protocol pipelines are defined by a declarative, routed graph of bindings, typically starting with a TCP server listening on a specific port.
Streams of data flow between Zilla bindings over shared memory. No matter the protocol, each stream has the same shape with a BEGIN frame to get started, followed by one or more DATA frames and an END or ABORT frame to complete the stream either normally or in error. The receiving side of a stream transfers WINDOW frames to the sender to manage back pressure via flow control, and potentially a RESET frame to tell the sender to stop abruptly. Streams also support a SIGNAL frame that can be delivered after a period of time has elapsed.

Protocols such as TCP are effectively raw binary data, so Zilla represents TCP over shared memory as a pair of streams, one in each direction with independent flow control and lifetime, making it straightforward to properly support half-closed TCP connections.
Zilla implements multiplexing protocols, such as HTTP/2, with a network side stream that decodes and encodes the raw protocol, and application side streams that represent higher level concepts, such as individual HTTP/2 request-response exchanges.
Although all Zilla streams have the same general shape, they also have protocol-specific extension metadata associated with one or more of the frame types flowing over shared memory. For example, the Zilla http binding represents application side streams with an HttpBeginEx extension attached to the BEGIN frame. The HttpBeginEx structure contains HTTP headers for the HTTP request or HTTP response stream. This application side HTTP request-response exchange stream representation is used for both HTTP/1.1 and HTTP/2 protocol streams, and later for HTTP/3 streams too.
Each protocol-specific binding can route conditionally to the next binding in the pipeline based on protocol-specific concepts. For example, the tcp binding can route by port, the tls binding can route by server name (SNI) or application layer protocol (ALPN), and the http binding can route by request headers.
Much like HTTP/2, Zilla implements MQTT with a network side stream that decodes and encodes the raw protocol, with application side streams that represent higher level concepts such as MQTT sessions, subscribers, and publishers. For example, the Zilla mqtt binding represents application side streams with a MqttBeginEx extension attached to the BEGIN frame.
Kafka defines a fairly complex wire protocol, both in terms of the fine-grained nature of the interactions due to the expectation of an intelligent client, and in terms of the evolution of the syntax of each different interaction. At the time of writing, Kafka wire protocol has 75 different fine-grained interactions (called API Keys) and several syntax versions of each one. Kafka clients are expected to make an initial connection to any broker in a Kafka cluster to discover how to connect to each different Kafka broker in the cluster as needed, depending on the topic partition where messages are being fetched or produced.
Zilla represents the Kafka protocol as application side streams for a subset of the fine-grained interactions as needed, such as fetch streams and produce streams for individual topic partitions, and a merged stream that represents an aggregated view of all partitions in a topic. For example, the Zilla kafka binding represents application side streams with a KafkaBeginEx extension attached to the BEGIN frame.
With these strongly typed yet similarly shaped stream abstractions in place for different protocols, mapping from one protocol to another simplifies mapping a combination of both the metadata present in the type-specific extensions and the lifetimes of the streams involved.

Zilla uses this simplified mapping approach to support HTTP, Server-Sent Events, gRPC and MQTT entry points to and from Kafka, such that the client can remain unaware that Kafka is present in the application architecture behind Zilla. Further, if the structure of message topics changes, or the location of the Kafka broker changes, the Zilla mapping can be adjusted as needed to continue to provide a consistent and compatible API to web, mobile, and IoT clients.
Scalability
Performance and scalability are related but distinct concepts. For example, performance can be the measurement of raw throughput or latency, whereas scalability is the measurement of how performance varies with increasing amounts of load and increasing amounts of available resources. Systems are said to be linearly scalable if they can handle additional load at a rate directly proportional to the amount of additional resources.
Vertical scaling refers to increasing the available resources on a single node, such as the memory, network, or the number of CPU cores, whereas horizontal scaling refers to increasing the number of nodes in the system to distribute the total load. Cross-core or cross-node coordination can negatively impact scalability to varying degrees.
Zilla is designed to help minimize such potential cross-core and cross-node coordination impact.
On startup, the Zilla engine parses its declarative configuration and creates a separate engine worker per CPU core. Each worker executes in its own single-threaded context and the modular concepts described above are also instantiated to execute in each single-threaded engine context. Therefore, when running on systems with more CPU cores, Zilla has more engine workers executing in parallel.
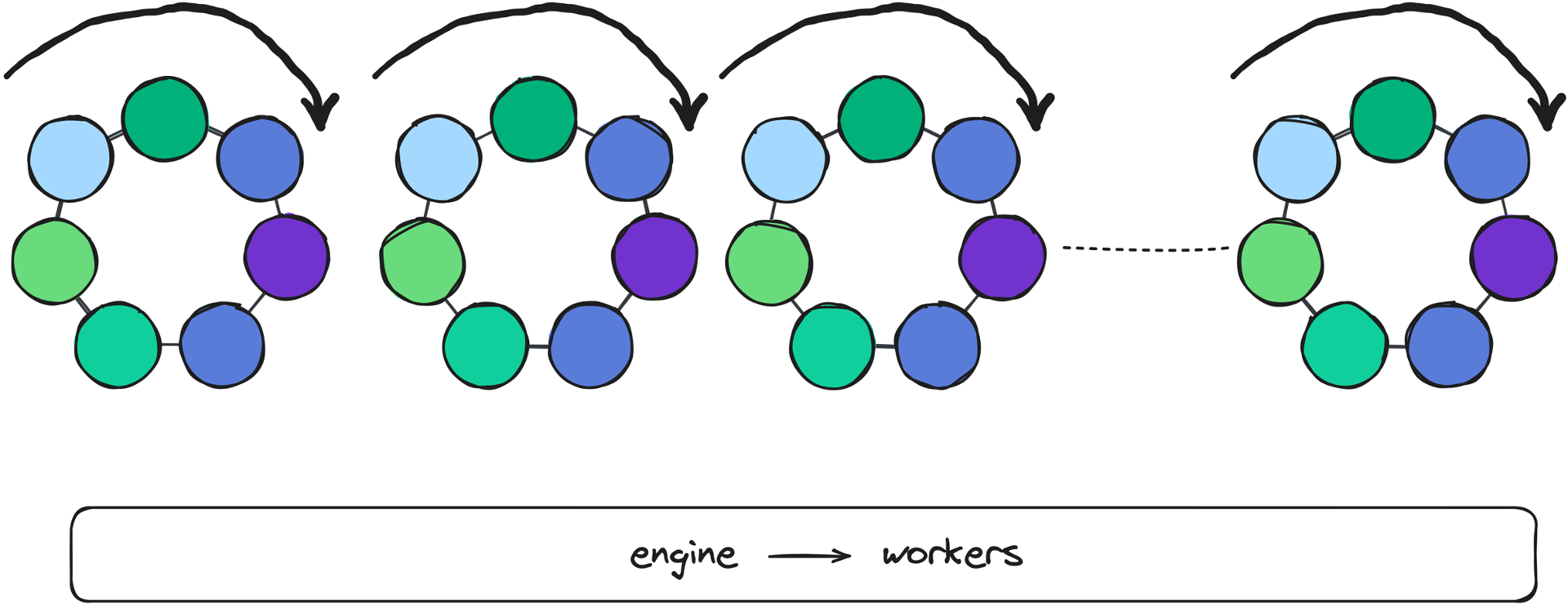
As clients connect to Zilla, each TCP connection is dispatched to one of the engine workers, where it progresses through the protocol pipeline on the same CPU core. The vast majority of Zilla engine worker execution has no contention across CPU cores, with the only exception for fan-in or fan-out scenarios.
When required to communicate across engine workers, Zilla uses lock-free data structures with ordered memory writes to minimize the coordination effort across CPU cores. Combining this with Zilla's shared memory stream backpressure via flow control allows all engine workers to work as fast as each receiver can consume their stream, making sure that one engine worker can never overwhelm another engine worker with too much data, while also eliminating unnecessary buffering that would otherwise incur additional effort to clear out the buffer.
Zilla minimizes the potential impact of Java garbage collection by avoiding unnecessary object allocation on the data path. All progress on shared memory streams is handled by reusing flyweight objects to access memory in a structured and strongly-typed manner. Zilla defines these typed structures declaratively and uses an internal tool to generate the corresponding flyweight code for each binding as needed.
A range of different scenarios can put different types of load on Zilla. For example, one scenario might comprise lots of clients with a relatively small amount of throughput per client, while another scenario might comprise relatively few clients with a significant amount of throughput per client, for about the same aggregate throughput overall. In practice, Zilla needs to be able to handle both of these scenarios, and anything in between, including scenarios that can change dynamically over time.
Therefore, Zilla isolates the TCP communication between the JavaVM and the operating system to the TCP binding. Each engine worker has a short thread stack, processing either a Java NIO socket channel operation signal or a shared memory stream frame, typically by writing a new frame to a shared memory stream. After the engine worker thread stack unwinds, it continues by reading the next Java NIO socket channel operation signal or the next shared memory stream frame. When there is nothing to process, the engine worker progressively backs off so that the operating system can let the CPU core idle as needed.
As a consequence of isolating the differences in number of client connections to the TCP binding, the performance and scalability characteristics of the various scenarios all look approximately the same in Zilla after the streams progress past the TCP binding in shared memory.
Zilla also prevents contention throughput stalls during the TLS handshake by executing CPU intensive math calculations on a secondary CPU core so that the engine worker is not blocked. TLS connections that have already completed their handshake can therefore continue to encrypt and decrypt their communication in parallel with new TLS connections that are mid-handshake. When the math calculations are complete, the secondary CPU core sends a SIGNAL frame to let the TLS connection continue with the handshake at the right moment. This optimization is isolated to the TLS binding, so all TLS-encrypted protocols such as HTTPS, MQTTS, etc can benefit from the same enhanced behavior.
Another way that the scenarios can vary is by message size. Some systems may be designed to favor lots of small messages or fewer large messages. When there is a mixture of message sizes, it is important that large messages not block the progress of smaller messages on other streams, even when processed by the same engine worker. Zilla handles this by internal message fragmentation, where DATA frames are split into smaller chunks to let DATA frames in other streams still make progress even before the larger message is completely transferred.
Zilla's approach to prefer same engine worker communication and lock-free data structures for cross engine worker communication both help to maximize the vertical scalability of adding more CPU cores. Isolating TCP communication to the TCP binding, short thread stacks, flyweights for strongly typed memory access to avoid garbage collection and message fragmentation all help to enhance the performance of each engine worker.
Zilla handles Kafka produce fan-in scenarios and Kafka fetch fan-out scenarios via the Kafka cache binding. The contents of the cache are hydrated from Kafka topic partitions, stored as memory-mapped files representing topic partition segments. Fetched messages are sent once from Kafka to Zilla over a small number of connections to Kafka, and served as needed by any client connected to Zilla. Produced messages are sent in batches as needed from Zilla to Kafka over a small number of connections to Kafka. The cache is spread across engine workers asymmetrically.
For example, the number of workers reading from the cache to support fan-out may be different than the number of engine workers writing to the cache. This allows cache readers to work in parallel with their topic partition cache writer to deliver messages to all interested fan-out clients across all engine workers.

The Kafka cache in Zilla understands and honors message retention policies defined by Apache Kafka, including message compaction. Filtering is also supported in Zilla by the Kafka cache, where new messages are indexed on entry to the cache for hardware-accelerated filtering checks made when clients read from the cache.
Handling such scenarios across multiple Zilla nodes does not incur additional coordination overhead at each Zilla node, so horizontal scalability is not impacted at Zilla. The additional impact is minimally felt at Kafka, which is hydrating each Zilla cache independently. However as mentioned above Kafka is really good at "dumb pipes", transferring the same messages to many different "intelligent clients".
Observability
Distributed systems can be notoriously difficult to diagnose because they typically require an observable view of causal events across the application architecture. Zilla has first-class local concepts of metrics, log events, and traces, plus exporter integrations to expose this telemetry as part of the global view provided by application monitoring systems, such as Prometheus and OpenTelemetry collectors.
Each engine worker has a memory-mapped circular buffer of stream frames representing a work queue to be processed. When processing a stream frame, it is common to write another stream frame into the work queue. For example, decoding a binary HTTP/2 frame on a network stream and propagating the payload to the application stream representing the request-response exchange. In most cases, the new stream frame is written to the same work queue for the same worker running on the same CPU core, while in some fan-in or fan-out scenarios, it is necessary to write to the work queue of a different engine worker running on a different CPU core.
Debugging such systems would normally require increasing the log-level verbosity to output more information, impacting functional behavior or performance, which is especially undesirable in production deployments. However, in a very real sense, Zilla is always running with an active binary formatted trace level log, because that's just how stream communication works within and across engine workers. By observing the aggregate view of all work queues across all engine workers, we can see a debuggable trace of behavior for each stream, formatted for consumption by humans or other systems as needed. In addition, the observable trace can be discovered passively and in parallel with engine worker processing, avoiding any unnecessary degradation in performance.
Metrics are currently recorded in a lightweight manner during stream processing but are also suitable for offloading to a secondary CPU core such that they are calculated passively with zero overhead during stream processing.
Log events are designed to communicate an unexpected interaction with an external system, such as an authentication failure to Kafka, that can be resolved by updating either the Zilla configuration or the external environment itself, depending on the root cause of mismatched expectations.
What's Next?
Although much of the initial emphasis has been justifiably centered on Apache Kafka so far, the extensible stream-based design of Zilla lends itself to integrating many different kinds of protocols.
No doubt we'll be mapping more protocols to Kafka, such as AMQP (JMS) and GraphQL, but Zilla is also well positioned for the evolution of messaging systems too, no matter what the future may hold.
Learn more about Zilla at https://docs.aklivity.io/zilla/ latest and join the community at https://www.aklivity.io/slack.